Using GPUs with Python
Questions
What is GPU acceleration?
How to enable GPUs (for instance with CUDA) in Python code?
How to deploy GPUs at HPC2N, UPPMAX, and LUNARC?
Objectives
Learn common schemes for GPU code acceleration
Learn about the GPU nodes at HPC2N, UPPMAX, and LUNARC
GPU-accelerated computing is when you use a graphics processing unit (GPU) along with a computer processing unit (CPU) to facilitate processing-intensive operations such as deep learning, analytics and engineering applications.
A GPU is a processor which is from many smaller and more specialized cores. When these cores work together, you can get a large performance boost for tasks that can be divided up and processed across many cores.
In a typical cluster, some GPUs are attached to a single node resulting in a CPU-GPU hybrid architecture. The CPU component is called the host and the GPU part the device.
We can characterize the CPU and GPU performance with two quantities: the latency and the throughput.
Latency refers to the time spent in a sole computation. Throughput denotes the number of computations that can be performed in parallel. Then, we can say that a CPU has low latency (able to do fast computations) but low throughput (only a few computations simultaneously). In the case of GPUs, the latency is high and the throughput is also high. We can visualize the behavior of the CPUs and GPUs with cars as in the figure below. A CPU would be compact road where only a few racing cars can drive whereas a GPU would be a broader road where plenty of slow cars can drive.
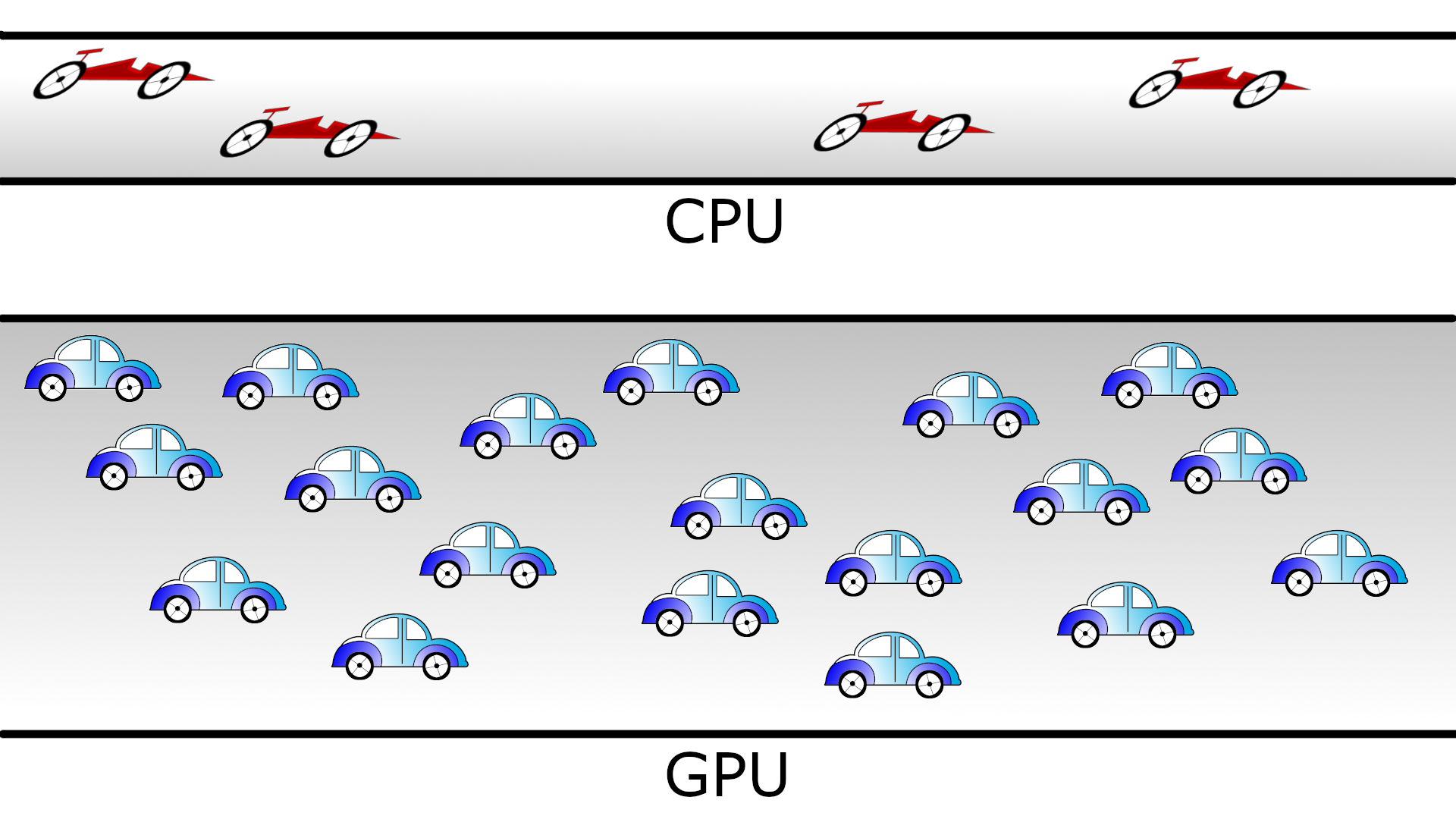
Cars and roads analogy for the CPU and GPU behavior. The compact road is analogous to the CPU (low latency, low throughput) and the broader road is analogous to the GPU (high latency, high throughput)
As an illustration a K80 GPU engine looks like this:
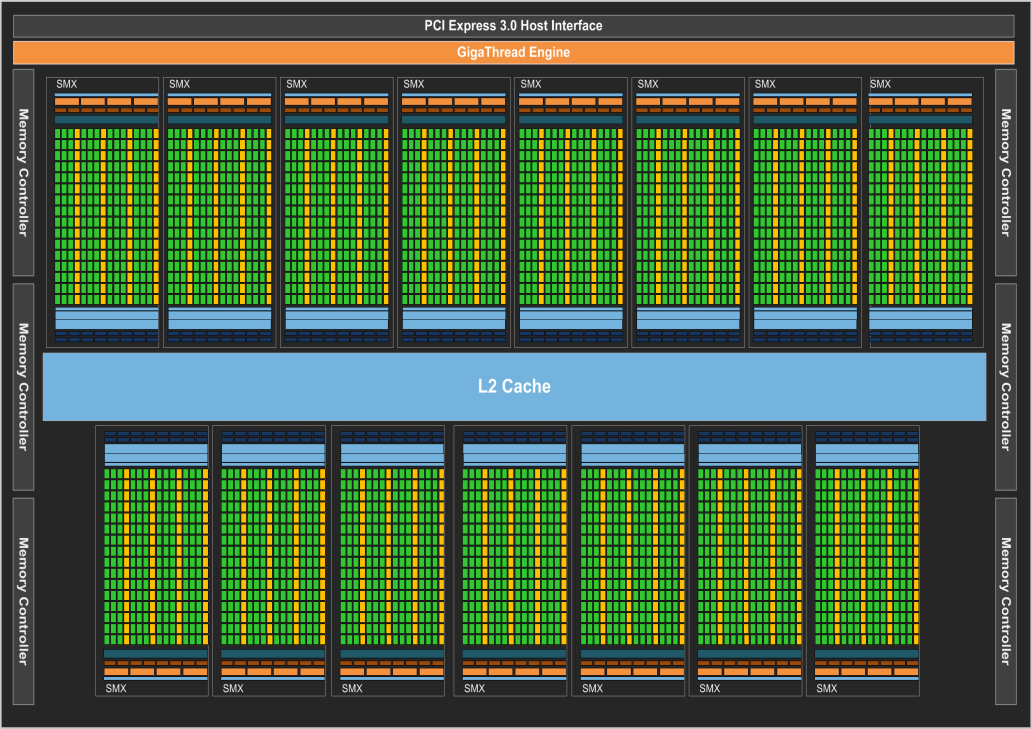
A single GPU engine of a K80 card. Each green dot represents a core (single precision) which runs at a frequency of 562 MHz. The cores are arranged in slots called streaming multiprocessors (SMX) in the figure. Cores in the same SMX share some local and fast cache memory.
In a typical cluster, some GPUs are attached to a single node resulting in a CPU-GPU hybrid architecture. The CPU component is called the host and the GPU part the device. One possible layout (Kebnekaise - the K80s are now retired) is as follows:
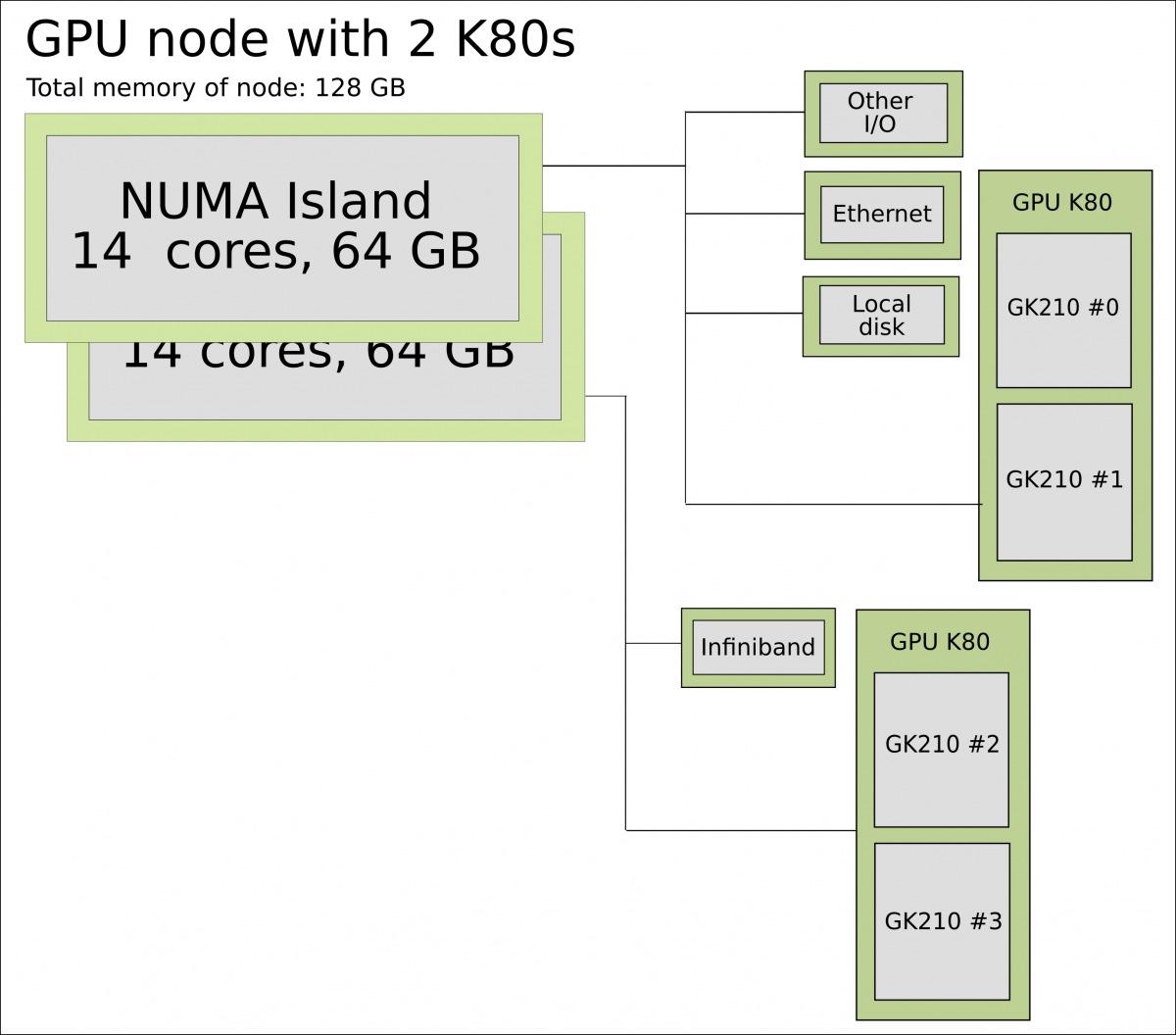
Schematics of a hybrid CPU-GPU architecture. A GPU K80 card consisting of two engines is attached to a NUMA island which in turn contains 14 cores. The NUMA island and the GPUs are connected through a PCI-E interconnect which makes the data transfer between both components rather slow.
Not every Python program is suitable for GPU acceleration. GPUs process simple functions rapidly, and are best suited for repetitive and highly-parallel computing tasks. GPUs were originally designed to render high-resolution images and video concurrently and fast, but since they can perform parallel operations on multiple sets of data, they are also often used for other, non-graphical tasks. Common uses are machine learning and scientific computation were the GPUs can take advantage of massive parallelism.
Many Python packages are not CUDA aware, but some have been written specifically with GPUs in mind. If you are usually working with for instance NumPy and SciPy, you could optimize your code for GPU computing by using CuPy which mimics most of the NumPy functions. Another option is using Numba, which has bindings to CUDA and lets you write CUDA kernels in Python yourself. This means you can use custom algorithms.
One of the most common use of GPUs with Python is for machine learning or deep learning. For these cases you would use something like Tensorflow or PyTorch libraries which can handle CPU and GPU processing internally without the programmer needing to do so.
GPUs on UPPMAX, HPC2N, and LUNARC systems
There are generally either not GPUs on the login nodes or they cannot be accessed for computations. To use them you need to either launch an interactive job or submit a batch job.
UPPMAX only
Rackham’s compute nodes do not have GPUs. You need to use Snowy for that. A useful module on Snowy is python_ML_packages/3.9.5-gpu
.
You need to use this batch command (for x being the number of cards, 1 or 2):
#SBATCH -M snowy
#SBATCH --gres=gpu:x
HPC2N
Kebnekaise’s GPU nodes are considered a separate resource, and the regular compute nodes do not have GPUs.
Kebnekaise has a great many different types of GPUs:
V100 (2 cards/node)
A40 (8 cards/node)
A6000 (2 cards/node)
L40s (2 or 6 cards/node)
A100 (2 cards/node)
H100 (4 cards/node)
MI100 (2 cards/node)
To access them, you need to use this to the batch system:
#SBATCH --gpus=x
where x is the number of GPU cards you want. Above are given how many are on each type, so you can ask for up to that number.
In addition, you need to add this to the batch system:
#SBATCH -C <type>
where type is
v100
a40
a6000
l40s
a100
h100
mi100
For more information, see HPC2N’s guide to the different parts of the batch system: https://docs.hpc2n.umu.se/documentation/batchsystem/resources/
LUNARC
LUNARC has Nvidia A100 GPUs and Nvidia A40 GPUs, but the latter ones are reserved for interactive graphics work on the on-demand system, and Slurm jobs should not be submitted to them.
Thus in order to use the A100 GPUs on Cosmos, add this to your batch script:
A100 GPUs on AMD nodes:
#SBATCH -p gpua100
#SBATCH --gres=gpu:1
These nodes are configured as exclusive access and will not be shared between users. User projects will be charged for the entire node (48 cores). A job on a node will also have access to all memory on the node.
A100 GPUs on Intel nodes:
#SBATCH -p gpua100i
#SBATCH --gres=gpu:<number>
where <number>
is 1 or 2 (Two of the nodes have 1 GPU and two have 2 GPUs).
Numba example
Numba is installed on HPC2N and LUNARC as a module. We also need numpy, so we are loading SciPy-bundle as we have done before. On UPPMAX numba is part of python_ML_packages, so we use that.
We are going to use the following program for testing (it was taken from a (now absent) linuxhint.com exercise but there are also many great examples at https://numba.readthedocs.io/en/stable/cuda/examples.html):
Python example add-list.py
using Numba
import numpy as np from timeit import default_timer as timer from numba import vectorize # This should be a substantially high value. NUM_ELEMENTS = 100000000 # This is the CPU version. def vector_add_cpu(a, b): c = np.zeros(NUM_ELEMENTS, dtype=np.float32) for i in range(NUM_ELEMENTS): c[i] = a[i] + b[i] return c # This is the GPU version. Note the @vectorize decorator. This tells # numba to turn this into a GPU vectorized function. @vectorize(["float32(float32, float32)"], target='cuda') def vector_add_gpu(a, b): return a + b; def main(): a_source = np.ones(NUM_ELEMENTS, dtype=np.float32) b_source = np.ones(NUM_ELEMENTS, dtype=np.float32) # Time the CPU function start = timer() vector_add_cpu(a_source, b_source) vector_add_cpu_time = timer() - start # Time the GPU function start = timer() vector_add_gpu(a_source, b_source) vector_add_gpu_time = timer() - start # Report times print("CPU function took %f seconds." % vector_add_cpu_time) print("GPU function took %f seconds." % vector_add_gpu_time) return 0 if __name__ == "__main__": main()
As before, we need the batch system to run the code. There are no GPUs on the login nodes.
Type-Along
$ interactive -A naiss2024-22-1202 -n 1 -M snowy --gres=gpu:1 -t 1:00:01 --gres=gpu:1 -t 1:00:01
You receive the high interactive priority.
Please, use no more than 8 GB of RAM.
salloc: Pending job allocation 9697978
salloc: job 9697978 queued and waiting for resources
salloc: job 9697978 has been allocated resources
salloc: Granted job allocation 9697978
salloc: Waiting for resource configuration
salloc: Nodes s195 are ready for job
_ _ ____ ____ __ __ _ __ __
| | | | _ \| _ \| \/ | / \ \ \/ / | System: s195
| | | | |_) | |_) | |\/| | / _ \ \ / | User: bbrydsoe
| |_| | __/| __/| | | |/ ___ \ / \ |
\___/|_| |_| |_| |_/_/ \_\/_/\_\ |
###############################################################################
User Guides: https://docs.uppmax.uu.se/
Write to support@uppmax.uu.se, if you have questions or comments.
[bbrydsoe@s195 python]$ ml uppmax python/3.11.8 python_ML_packages/3.11.8-gpu
[bbrydsoe@s195 python]$ python add-list.py
CPU function took 35.272032 seconds.
GPU function took 1.324215 seconds.
Running a GPU Python code interactively.
$ salloc -A hpc2n2024-114 --time=00:30:00 -n 1 --gpus=1 -C a100
salloc: Pending job allocation 29039771
salloc: job 29039771 queued and waiting for resources
salloc: job 29039771 has been allocated resources
salloc: Granted job allocation 29039771
salloc: Waiting for resource configuration
salloc: Nodes b-cn1610 are ready for job
$
$ module load GCC/12.3.0 OpenMPI/4.1.5 numba/0.58.1 SciPy-bundle/2023.07 CUDA/12.0.0
$ srun python add-list.py
CPU function took 19.601633 seconds.
GPU function took 0.194873 seconds.
Batch script, “add-list-kebnekaise.sh”, to run the same GPU Python script (the numba code, “add-list.py”) at Kebnekaise. As before, submit with “sbatch add-list-kebnekaise.sh” (assuming you called the batch script thus - change to fit your own naming style).
#!/bin/bash
# Remember to change this to your own project ID after the course!
#SBATCH -A hpc2n2024-114
# We are asking for 5 minutes
#SBATCH --time=00:05:00
# Asking for one A100 GPU
#SBATCH --gpus=1
#SBATCH -C a100
# Remove any loaded modules and load the ones we need
module purge > /dev/null 2>&1
module load GCC/12.3.0 OpenMPI/4.1.5 numba/0.58.1 SciPy-bundle/2023.07 CUDA/12.0.0
# Run your Python script
python add-list.py
Batch script, “add-list-cosmos.sh”, to run the same GPU Python script (the numba code, “add-list-cosmos.py”) at Cosmos. As before, submit with “sbatch add-list-cosmos.sh” (assuming you called the batch script thus - change to fit your own naming style).
#!/bin/bash
# Remember to change this to your own project ID after the course!
#SBATCH -A lu2024-7-80
# We are asking for 5 minutes
#SBATCH --time=00:05:00
#SBATCH --ntasks-per-node=1
# Asking for one A100 GPU
#SBATCH -p gpua100
#SBATCH --gres=gpu:1
# Remove any loaded modules and load the ones we need
module purge > /dev/null 2>&1
module load GCC/12.2.0 OpenMPI/4.1.4 numba/0.58.0 SciPy-bundle/2023.02
# Run your Python script
python add-list.py
Exercises
Integration 2D with Numba
An initial implementation of the 2D integration problem with the CUDA support for Numba could be as follows:
integration2d_gpu.py
from __future__ import division
from numba import cuda, float32
import numpy
import math
from time import perf_counter
# grid size
n = 100*1024
threadsPerBlock = 16
blocksPerGrid = int((n+threadsPerBlock-1)/threadsPerBlock)
# interval size (same for X and Y)
h = math.pi / float(n)
@cuda.jit
def dotprod(C):
tid = cuda.threadIdx.x + cuda.blockIdx.x * cuda.blockDim.x
if tid >= n:
return
#cummulative variable
mysum = 0.0
# fine-grain integration in the X axis
x = h * (tid + 0.5)
# regular integration in the Y axis
for j in range(n):
y = h * (j + 0.5)
mysum += math.sin(x + y)
C[tid] = mysum
# array for collecting partial sums on the device
C_global_mem = cuda.device_array((n),dtype=numpy.float32)
starttime = perf_counter()
dotprod[blocksPerGrid,threadsPerBlock](C_global_mem)
res = C_global_mem.copy_to_host()
integral = h**2 * sum(res)
endtime = perf_counter()
print("Integral value is %e, Error is %e" % (integral, abs(integral - 0.0)))
print("Time spent: %.2f sec" % (endtime-starttime))
Notice the larger size of the grid in the present case (100*1024) compared to the serial case’s size we used previously (10000). Large computations are necessary on the GPUs to get the benefits of this architecture.
One can take advantage of the shared memory in a thread block to write faster
code. Here, we wrote the 2D integration example from the previous section where
threads in a block write on a shared[] array. Then, this array is reduced
(values added) and the output is collected in the array C
. The entire code
is here:
integration2d_gpu_shared.py
from __future__ import division
from numba import cuda, float32
import numpy
import math
from time import perf_counter
# grid size
n = 100*1024
threadsPerBlock = 16
blocksPerGrid = int((n+threadsPerBlock-1)/threadsPerBlock)
# interval size (same for X and Y)
h = math.pi / float(n)
@cuda.jit
def dotprod(C):
# using the shared memory in the thread block
shared = cuda.shared.array(shape=(threadsPerBlock), dtype=float32)
tid = cuda.threadIdx.x + cuda.blockIdx.x * cuda.blockDim.x
shrIndx = cuda.threadIdx.x
if tid >= n:
return
#cummulative variable
mysum = 0.0
# fine-grain integration in the X axis
x = h * (tid + 0.5)
# regular integration in the Y axis
for j in range(n):
y = h * (j + 0.5)
mysum += math.sin(x + y)
shared[shrIndx] = mysum
cuda.syncthreads()
# reduction for the whole thread block
s = 1
while s < cuda.blockDim.x:
if shrIndx % (2*s) == 0:
shared[shrIndx] += shared[shrIndx + s]
s *= 2
cuda.syncthreads()
# collecting the reduced value in the C array
if shrIndx == 0:
C[cuda.blockIdx.x] = shared[0]
# array for collecting partial sums on the device
C_global_mem = cuda.device_array((blocksPerGrid),dtype=numpy.float32)
starttime = perf_counter()
dotprod[blocksPerGrid,threadsPerBlock](C_global_mem)
res = C_global_mem.copy_to_host()
integral = h**2 * sum(res)
endtime = perf_counter()
print("Integral value is %e, Error is %e" % (integral, abs(integral - 0.0)))
print("Time spent: %.2f sec" % (endtime-starttime))
Prepare a batch script to run these two versions of the integration 2D with Numba support and monitor the timings for both cases.
Here follows a solution for HPC2N. Try and make it run on Snowy, using the python_ML_packages for Python 3.11.8 and the changes suggested by the UPPMAX solution for add-list.py above.
Solution for HPC2N
A template for running the python codes at HPC2N is here:
integration2d_gpu.sh
#!/bin/bash # Remember to change this to your own project ID after the course! #SBATCH -A hpc2n2024-114 #SBATCH -t 00:08:00 #SBATCH -N 1 #SBATCH -n 28 #SBATCH -o output_%j.out # output file #SBATCH -e error_%j.err # error messages #SBATCH --gpus=1 #SBATCH -C a100 ml purge > /dev/null 2>&1 module load GCC/12.3.0 OpenMPI/4.1.5 numba/0.58.1 SciPy-bundle/2023.07 CUDA/12.0.0 python integration2d_gpu.py python integration2d_gpu_shared.pyFor the
integration2d_gpu.py
implementation, the time for executing the kernel and doing some postprocessing to the outputs (copying the C array and doing a reduction) was 4.35 sec. which is a much smaller value than the time for the serial numba code of 152 sec obtained previously.The simulation time for the
integration2d_shared.py
implementation was 1.87 sec. by using the shared memory trick.
Keypoints
You deploy GPU nodes via SLURM, either in interactive mode or batch
In Python the numba package is handy
Additional information
Hands-On GPU Programming with Python and CUDA : Explore High-Performance Parallel Computing with CUDA, Brian Tuomanen. Packt publishing.
Parallel and High Performance Computing, Robert Robey and Yuliana Zamora. Manning publishing.